Strategic-Human to AI-Tactical Performance
- by Tallgrass
- May 29, 2020
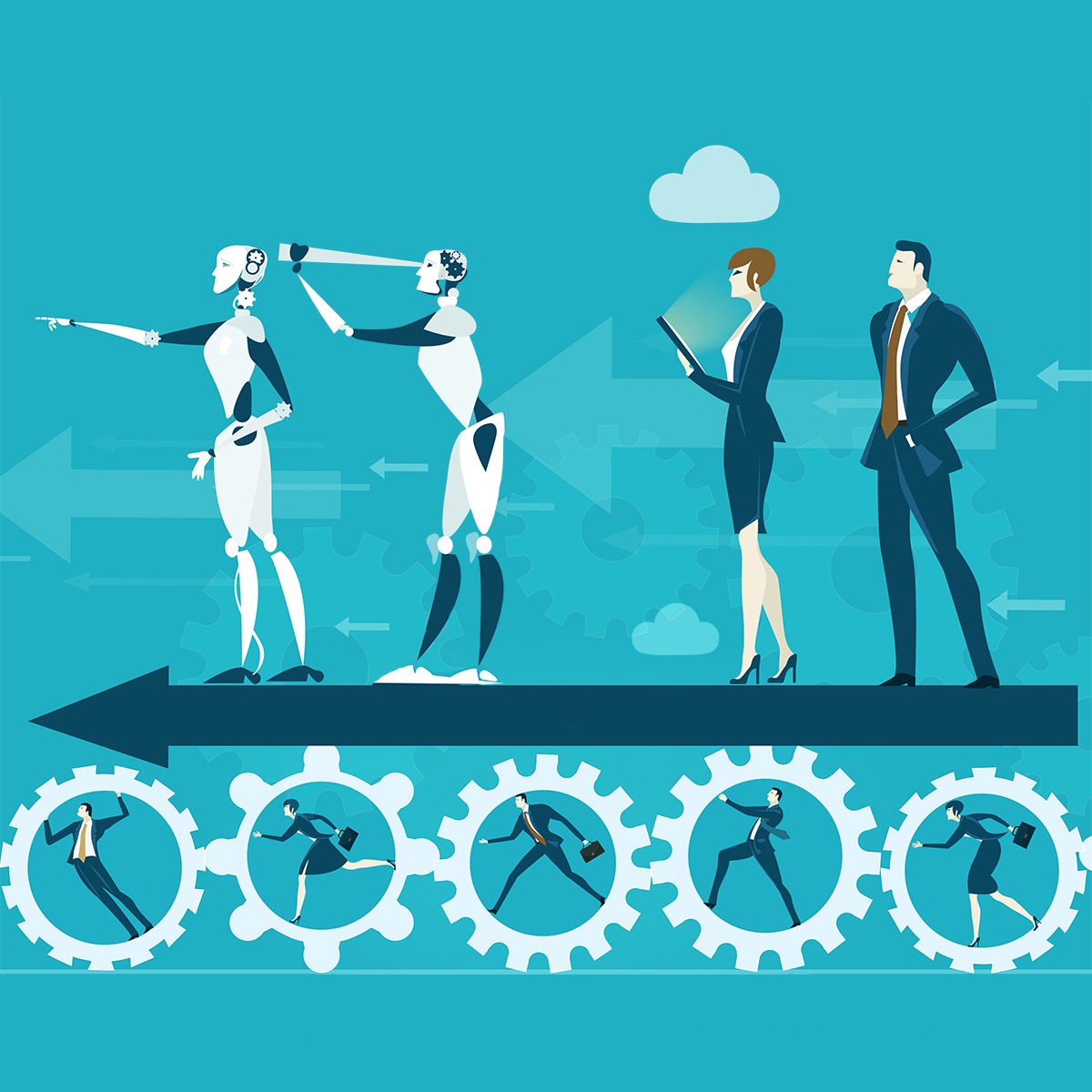
The performance of measuring processes and even AI decisions specific to strategic decision support is the future of the modern decision support systems.
Impact Analysis is a method to relate human decisions to intelligent processes based on Operational Failure, or OpFailure. OpFailure is human-understandable and is defined within each model just as feature sets are within machine learning and AI.
The design of Failure is achieved within the initial steps of Impact Analysis and is comprised of simple statements easily understood and acted upon by decision-makers and engineers. An instance or occurrence of OpFailure by itself is benign yet observable over time and magnitude. Most importantly human understanding of what an OpFailure is can be aligned more closely to AI’s optimal outcomes.
For instance, the times a product is ordered but is out of stock. We might define OpFailure as the times this occurred over a period of time that amounted to greater than a set amount of revenue. The times and amount would be configured so that if it occurred once we could safely ignore it but as the count increased seek to observe any velocity of change. In our example; say we measured 1% of a given product is responsible for 15% of all OpFailure.
The variance between 15% and 1% is what we call “impact,” and in this instance, it would be overstated by 14% and ordered higher than all other products. Within the processes of Impact Analysis, the velocity of OpFailure changing over time is measured per product, as well as the overall impact between them all. As a product’s percentage of OpFailure changes over time, it moves through bands of performance levels from observable pre-failure to actionable failure. Outside of identifying the growing performance issue, there is an opportunity to visually observe where minimal effort would have the greatest benefit and further enrich our Artificial Intelligence-driven feature set – as well as issue prescribed edicts to correct them.
By combining OpFailure as a feature within Machine Learning we can pursue independent predictability and optimize occurrences. This allows us to fail smaller and sooner in an observable and predictable manner yet have the actionable means to surgically apply prescribed edicts when needed. Once an OpFailure is defined its behavior is captured and applied against historical data with the help of the BAS. (Behavioral Analysis System) The BAS is a next-generation back-testing technology that splits data into sets via performance bands and the magnitude of OpFailure. Once seeded, history is “replayed” or back-tested into the sets across global average/prefailure/failure/compliance.
Now the data-segmented Impact Analysis has the ideal information to illustrate inequalities found where equal efforts do not produce equal outcomes. In the following examples, we illustrate a time series variance that indicates the velocity of averaged change > current in a timeline running “horizontally” as a horizontal impact. Below that we review what a vertical impact is as well.
INTRODUCING IMPACT ANALYSIS
Again, Impact Analysis orders the universe by performance based on OpFailure. Opportunity can be plotted over time to illustrate elements with the high velocity of change against its current performance where variance and impact are calculated and ordered. (Horizontally) Opportunity can also be plotted to compare element performance to other elements showing inequalities. (Vertically) Both types of Impact Analysis can include many measures, elements, or dimensions like date, product type, and geographic location. Each dimension is intersected with all others allowing the illustrations to pivot AI-based outcomes and reveal the related AI performance.
Through Impact Analysis decision-makers can visually command an unlimited data footprint and easily relate the performance of intelligent processes to their decisions. As some performance bands are designed to be observable, others are actionable. Actionable bands have a lower threshold of performance and when triggered intervening commands are prescribed that correct or require a process to deviate. For every prescribed intervention the means to measure compliance is determined beforehand. By manufacturing bands of performance, we can now isolate and measure improved outcomes and even monetized compliance. This concept can highly benefit business decision making of AI performance.
Two types of impacts:
- Time-based (a period is averaged for a given metric, variance and impact calculated then ordered by performance) = measuring a degree of performance difference between the current period compared to the rolling average. Rolling average 50, Current 60, Variance, and Impact +10 for a given item.
- Metric based (performance is impacted by items within its common group against two independent variables or performance bands) = measures a degree of performance difference between like items. For a given item type, has a contribution percentage of 50% but a contribution of failure count of 60%, it has variance and impact of +10%
Example – Time Based Impact
Traditionally Time Based Impacts are visualized horizontally and ordered to identify the greatest growing velocity of change of past to current performance of a single item, node, or element to itself.
The velocity of change is calculated via a rolling weighted average, here across 8 months. Next, the current metric is plotted and the rolling average subtracted yielding our impact. The grid is then sorted within the IMPACT column so the performance with the greatest positive and negative impact bubbles to the top and bottom.
Figure 1, Illustration of a horizontal time-based impact complete with alerts for the top items that show the greatest inequality and velocity of change in performance.
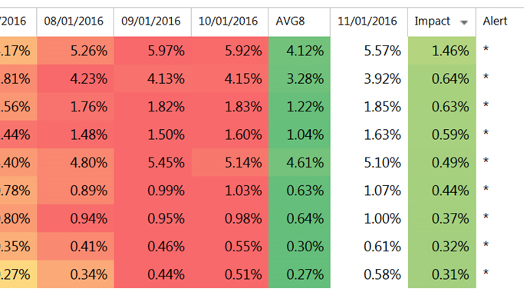
Example – Metric Based Impact
Traditionally Metric Based Impacts are visualized vertically and ordered to identify the greatest difference of an item’s contribution compared to another measure. This one of the prominent decision-making techniques that allow users to focus on the greatest inequality, for example, item # VSAR is 3.84% of the universe yet equates to 8.35% of activity, it has inequality of 4.51% See Figure 2 below.
Figure 2, illustration a vertical metric based impact.
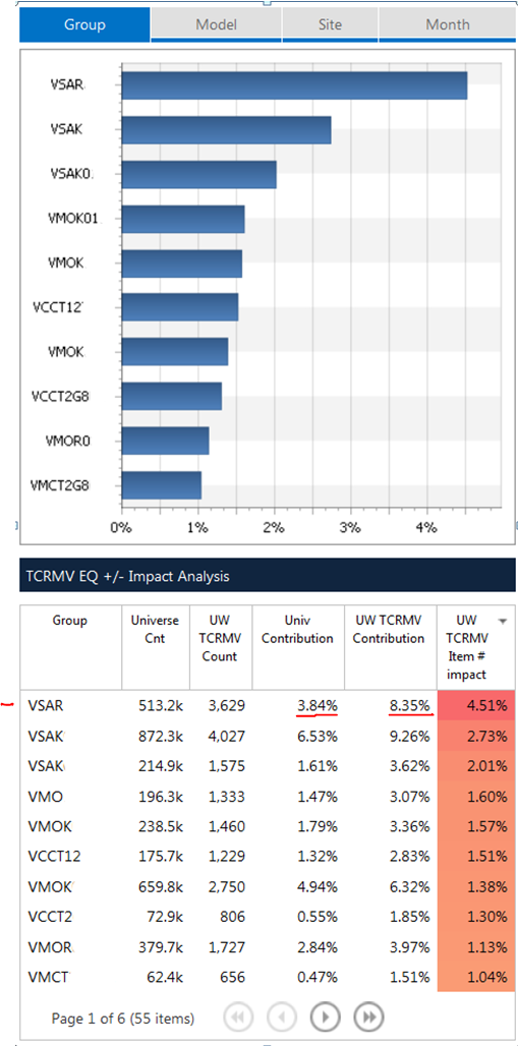
Example – metric based impact continued
right track posting a negative overall impact for the last month at -1.59. Note, if the impact column was summed it will always equal 0. Where an item falls to the left or right of 0 is what provides us with the inequality of one measure to another.
Figure 3, metric-based vertical impact continued.
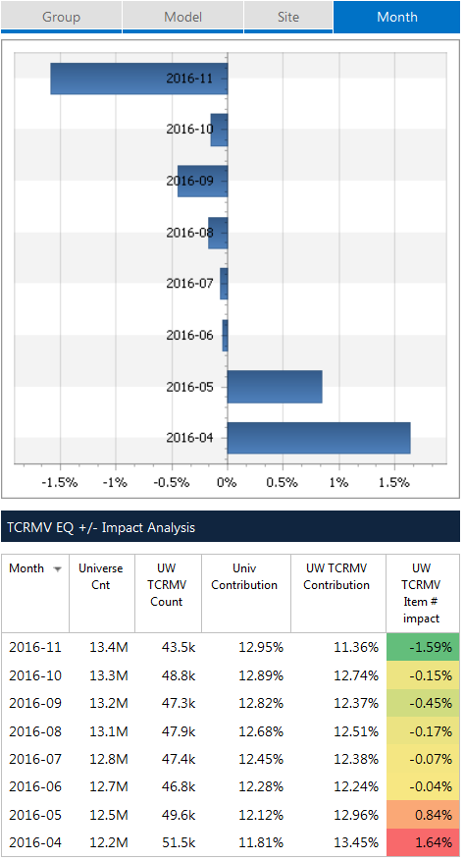
Conclusion
The previous charts provide the lessons learned on the power of impact analysis. Overall the entire dataset reduced this behavior from 13.45 to 11.36 in seven weeks. In the illustration, we are clearly making progress but will not reach our goal unless we can understand what single items have the greatest impact and how to influence and track progress. Here they are visible and actionable.
Impact Analysis naturally orders information by the most impactful items. As human and AI decisions are made to optimize one process its positive impact will eventually be stopped by weaker processes.
The decision support model and the role of artificial intelligence in business decision making requires its leaders to understand and how to measure these states and how to respond.
Since it was possible to train executive decision-makers to work through scorecards and down into the drivers of performance a reusable framework was developed and greater input and ownership of metrics achieved.
One Reply to “Strategic-Human to AI-Tactical Performance”
Major thankies for the article post. Really looking forward to read more. Great. Mildred Des Cramer